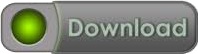
The coefficients of the variables of interest are not affected, and the performance of the control variables as controls is not impaired. Here’s an example from some of my own work: the sample consists of U.S. colleges, the dependent variable is graduation rate, and the variable of interest is an indicator (dummy) for public vs. Two control variables are average SAT scores and average ACT scores for entering freshmen. These two variables have a correlation above. 9, which corresponds to VIFs of at least 5.26 for each of them. But the VIF for the public/private indicator is only 1.04. So there’s no problem to be concerned about, and no need to delete one or the other of the two controls.Ģ. The high VIFs are caused by the inclusion of powers or products of other variables. If you specify a regression model with both x and x 2, there’s a good chance that those two variables will be highly correlated. Similarly, if your model has x, z, and xz, both x and z are likely to be highly correlated with their product.
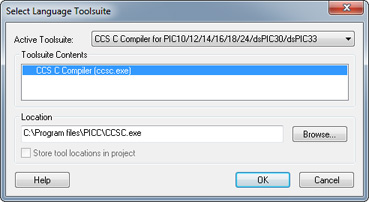
This is not something to be concerned about, however, because the p-value for xz is not affected by the multicollinearity. This is easily demonstrated: you can greatly reduce the correlations by “centering” the variables (i.e., subtracting their means) before creating the powers or the products. But the p-value for x 2 or for xz will be exactly the same, regardless of whether or not you center. And all the results for the other variables (including the R 2 but not including the lower-order terms) will be the same in either case. So the multicollinearity has no adverse consequences.ģ. The variables with high VIFs are indicator (dummy) variables that represent a categorical variable with three or more categories.
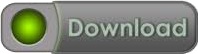